Monday (01.13)
์ค๋์ ์ ์ฌ
๋ณ์ โญ๏ธ โญ๏ธ โญ๏ธ
๊ตฌ๋ด์๋น์์ ๋จน์๋ค.
5500์... ๊ทธ๋ฅ ๋จน์
๋ค์ด๋ฒ ์ง๋
DMC์ฒจ๋จ์ฐ์ ์ผํฐ
map.naver.com
์ ๋ณด์ฒ๋ฆฌ๊ธฐ์ฌ ํ๊ธฐ ์ํ ์ ์ฒญ
14์์ ์๊ฐ์ ์ฒญ์ฒ๋ผ ์ค๋นํด์ ์ ์ฒญ ํ์๋ค.
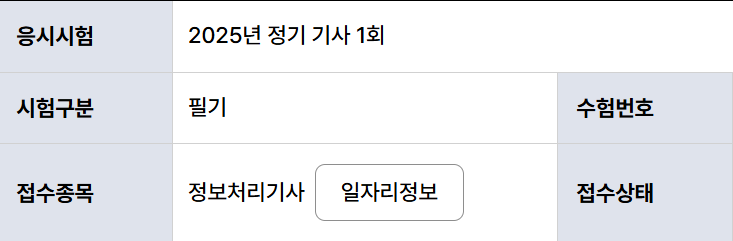
CS ๊ณต๋ถํ ๋ ํ ์คํธ๋ฅผ ์ํด 4ํ์ ๋ ํ์ด๋ดค์๋๋ฐ, ๋จ์ ์๊ธฐ ๋ฌธ์ ๋นผ๊ณ ๋ ํ๋ง ํ๊ฒ ๊ฐ์์, "์์ผ๋ฉด ์ข๊ณ " ๋ผ๋ ๋ง์ธ๋๋ก ์ํ์ ์ณ ๋ณด๋ ค๊ณ ํ๋ค.
Adsp๋ ๋ด์ผํด์ ์๊ฐ์ด ๊ฒน์น๊ธด ํ์ง๋ง, ์ถฉ๋ถํ ๋ณผ ์ ์์ ๊ฒ ๊ฐ๋ค. ๋ฐ์๊ฒ ์ด์
์ค๋์ EDA์ ๋ํ ์์ ์งํ
Exploratory Data Analysis (EDA)
๋ฐ์ดํฐ๋ฅผ ์ฒ์ ์ ํ ๋ ๊ทธ ํน์ฑ์ ํ์ํ๋ ๊ณผ์ ์ผ๋ก, ๋ฐ์ดํฐ๋ฅผ ์ดํดํ๊ณ ๋ ๋์ ๋ถ์์ ์ํด ์ค๋นํ๋ ๋จ๊ณ
๊ธฐ์ ํต๊ณ๋ ์๊ฐํ ๋ฐฉ๋ฒ์ ํ์ฉํ์ฌ ๋ฐ์ดํฐ๋ฅผ ๋ค๊ฐ๋๋ก ๋ถ์ํ๊ณ ๋ฌธ์ ๋ฅผ ์ ์ํ๋ ๋ฐ ์ค์ํ ์ญํ
- ํต๊ณํ: ์์ง๋ ์๋ฃ์ ๋ํ ๋ถ์ → ๊ณผ๊ฑฐ
- ๋จธ์ ๋ฌ๋: ๋ค์ด์ฌ ์๋ฃ์ ๋ํ ์ถ๋ก → ๋ฏธ๋
์์๋ฐ์ดํฐ๋ฅผ ๊ฐ์ง๊ณ ๋ฐ์ดํฐ์ ํน์ง,๊ตฌ์กฐ๋ก๋ถํฐ ์ ๋ณด๋ฅผ ์ป์ด์ ์ด๋ฅผ ๋ฐํ์ผ๋ก ํต๊ณ๋ชจํ์ ๋ง๋๋ ๋ถ์๋ฐฉ๋ฒ
๋ชฐ๋๋ ์ฃผ์ ์ฉ์ด
- ์ผํ์ ์ฆ๊ฑฐ (Anecdotal Evidence): ๊ณผํ์ ์ฐ๊ตฌ์ ์ํด ์์ง๋์ง ์์, ์ฐ์ฐํ ์ป์ ์ฆ๊ฑฐ
- ๋จ์ ์ฐ๊ตฌ (Cross-sectional Study): ํน์ ์์ ์ ๋ฐ์ดํฐ๋ฅผ ์์งํ๋ ์ฐ๊ตฌ
- ํก๋จ์ ์ฐ๊ตฌ (Longitudinal Study): ์๊ฐ์ ๋ฐ๋ผ ๋ฐ์ดํฐ๋ฅผ ์ถ์ ํ๋ฉฐ ๋ฐ๋ณต์ ์ผ๋ก ์์งํ๋ ์ฐ๊ตฌ
- ์ค๋ฒ์ํ๋ง (Oversampling): ํ๋ณธ ํฌ๊ธฐ๊ฐ ์์ ๊ฒฝ์ฐ ์ค๋ฅ๋ฅผ ๋ฐฉ์งํ๊ธฐ ์ํด ์ฌ์ฉํ๋ ๋ฐฉ๋ฒ
- ์ฌ์ฝ๋ (Recode): ๋ฐ์ดํฐ๋ฅผ ๋ค๋ฅธ ๋ฐฉ์์ผ๋ก ์์ ํ๊ฑฐ๋ ๊ณ์ฐํ์ฌ ์๋ก์ด ๋ฐ์ดํฐ๋ฅผ ์์ฑํ๋ ๊ณผ์
- ์๋ฃ ์ ์ (Data Cleaning): ๋ฐ์ดํฐ์ ์ค๋ฅ๋ฅผ ์์ ํ๊ณ , ์ ์ ํ ํํ๋ก ๋ณํํ์ฌ ๋ถ์์ ์ ํฉํ ๋ฐ์ดํฐ๋ฅผ ๋ง๋๋ ๊ณผ์
EDA ํ๋ก์ธ์ค
- ๋ฌธ์ ์ ์: ๋ถ์์ ๋ชฉํ์ ๋ฌธ์ ๋ฅผ ๋ช ํํ ์ค์
- ๋ฐ์ดํฐ ์์ง: ๋ฌธ์ ํด๊ฒฐ์ ํ์ํ ๋ฐ์ดํฐ ์์ง
- ๋ฐ์ดํฐ ์ ์ฒ๋ฆฌ: ๋ฐ์ดํฐ๋ฅผ ์ ๋ฆฌํ๊ณ , ๊ฒฐ์ธก๊ฐ ์ฒ๋ฆฌ, ์ด์์น ํ์ง ์ํ
- ๋ฐ์ดํฐ ๋ชจ๋ธ๋ง: ๋ฐ์ดํฐ๋ฅผ ๋ฐํ์ผ๋ก ๋ชจ๋ธ์ ๋ง๋ค๊ณ ๋ถ์
- ์๊ฐํ ๋ฐ ํ์: ๋ฐ์ดํฐ์ ํจํด์ ์๊ฐ์ ์ผ๋ก ํ์
EDA๋ ์ด 5๋จ๊ณ๋ฅผ ํ ๋ฒ์ ์๋ฃํ๋ ๊ฒ์ด ์๋๋ผ, ๋ถ์กฑํ ๋จ๊ณ๊ฐ ์์ผ๋ฉด ๋ฐ๋ณต์ ์ผ๋ก ๋์๊ฐ๋ฉด์ ๊ฐ์ ํด๋๊ฐ๋ ๋ฐฉ์์ผ๋ก ์งํ
ํ์ฆ์ ๋ฐ์ดํฐ ๋ถ์ (CDA) vs. ํ์์ ๋ฐ์ดํฐ ๋ถ์ (EDA)
- ํ์ฆ์ ๋ฐ์ดํฐ ๋ถ์ (CDA): ๊ธฐ์กด์ ์ค์ ํ ๊ฐ์ค์ ๊ฒ์ฆํ๋ ๋ถ์ ๋ฐฉ๋ฒ ๊ฐ์ค์ ์ค์ ํ๊ณ ๋ฐ์ดํฐ๋ฅผ ์์งํ์ฌ ์ถ์ ํ๊ฑฐ๋ ํ๊ฐํ๋ ๋ฐฉ๋ฒ(์ถ๋ก ํต๊ณ)
- ํ์์ ๋ฐ์ดํฐ ๋ถ์ (EDA): ๋ฐ์ดํฐ๋ฅผ ์ฒ์๋ถํฐ ๋๊น์ง ํ์ํ์ฌ ํจํด์ด๋ ๊ด๊ณ๋ฅผ ๋ฐ๊ฒฌํ๋ ๋ถ์ ๋ฐฉ๋ฒ(๊ธฐ์ ํต๊ณ)
- ๋น ๋ฐ์ดํฐ ๋ชจ๋ธ๋ง: ์๋ก ๋ค์ด์ค๋ ๋ฐ์ดํฐ๋ฅผ ์ผ๋ง๋ ์ ์ค๋ช ํ ์ ์๋์ง๊ฐ ์ค์ํ ๋ชฉํ -> ๊ธฐ์ ํต๊ณ๊ฐ ๋น ๋ฐ์ดํฐ ๋ถ์์ ์ ํฉ
๊ณ ๋ คํด์ผ ํ ์
- ์๊ด๊ด๊ณ vs. ์ธ๊ณผ๊ด๊ณ: ์๊ด๊ด๊ณ๊ฐ ๋ฐ๋์ ์ธ๊ณผ๊ด๊ณ๋ฅผ ์๋ฏธํ์ง ์์
- ์๊ด๊ด๊ณ๋ ๋ ๋ณ์ ๊ฐ์ ๊ด๊ณ๋ฅผ ๋ณด์ฌ์ฃผ์ง๋ง, ํญ์ ์ธ๊ณผ๊ด๊ณ๋ฅผ ์๋ฏธํ์ง ์์ผ๋ฏ๋ก ์ด๋ฅผ ๊ตฌ๋ถํ์ฌ ๋ถ์ํด์ผ ํจ
- ์ด์์น ํ์ง: ๋ถ์์์ ์ค์ํ ์์๋ก, ์ด์์น๋ ๊ฒฐ๊ณผ์ ํฐ ์ํฅ ๋ฏธ์น ์ ์์
- 3์ฐจ์ ์๊ฐํ๋ ์๊ธ๊ฐ์ผ๋ก ์ธ์ง์ ๋ํ ์ค์ฐจ๊ฐ ์๊ธฐ๋ ๋ฌธ์ ์กด์ฌ. ๋ฏธ์ ์๋ฆ๋ค์๋ณด๋ค ๋ฐ์ดํฐ ์ดํด์ ๊ฑธ๋ฆฌ๋ ์๊ฐ๋จ์ถ์ ์ค์
- ๋์๋ณด๋ : ๋ค์ํ ๋ฐ์ดํฐ๋ฅผ ๋์์ ๋น๊ตํ ์ ์๊ฒ ํด ์ฃผ๋ ์ฌ๋ฌ ๋ทฐ์ ๋ชจ์
๋ฐ์ดํฐ์ ์ข
๋ฅ์ ๋ฐ๋ฅธ ๋ถ๋ฅ
- ์ง์ ๋ณ์: ๋ช
๋ชฉํ(์์์ ์๋ฏธ๋ถ์ฌ ๋ถ๊ฐ), ์์ํ(์์์ ์๋ฏธ๋ถ์ฌ ๊ฐ๋ฅ)
- ๋ช ๋ชฉํ : ์ฑ๋ณ, ํ์กํ, ์ง์ญ
- ์์ํ : ํ๋ , ๊ณ ๊ฐ๋ฑ๊ธ
- ์์ ๋ณ์: ์ด์ฐํ, ์ฐ์ํ
- ์ด์ฐํ : ํ์ ์, ์ฃผ๋ฌธ ์
- ์ฐ์ํ: ๋ชธ๋ฌด๊ฒ, ํค
๋ฐ์ดํฐ ์๊ฐํ
- ์๊ฐ ์๊ฐํ: ์๊ณ์ด ๋ฐ์ดํฐ๋ฅผ ์๊ฐ์ ์ผ๋ก ํํํ์ฌ ์๊ฐ์ ๋ฐ๋ฅธ ๋ณํ
- ์๊ณ์ด ๋ฐ์ดํฐ: ์๊ฐ ์์์ ๋ฐ๋ฅธ ๋ฐ์ดํฐ
- ๋ถ์ ํ ๋ฐ์ดํฐ: ์ด์ฐํ ๋ฐ์ดํฐ๋ก ํน์ ์์ ์ ๋ํ๋
- ๋ถํฌ ์๊ฐํ
- ์๊ทธ๋ํ: ์ ์ ์์ ํญ๋ชฉ์ ํผ์ผํธ์ ํจ๊ป ๋ณด์ฌ์ฃผ๋ ๊ฒ์ด ์ข์
- ํธ๋ฆฌ๋งต: ์์ญ ๊ธฐ๋ฐ ์๊ฐํ,๊ณ์ธต์ ์ธ ๊ตฌ์กฐ๋ฅผ ํํํ ๋ ์ฌ์ฉ
- ๋์ ์ฐ์ ๊ทธ๋ํ: ์๊ณ์ด ๋ฐ์ดํฐ๋ฅผ ๋์ ํ์ฌ ํํํ ๋ ์ฌ์ฉ
- ๊ด๊ณ ์๊ฐํ
- ์ฐ์ ๋ (Scatter Plot): ๋ ๋ณ์ ๊ฐ์ ๊ด๊ณ๋ฅผ ์๊ฐ์ ์ผ๋ก ๋ํ๋. ์์ ์๊ด๊ด๊ณ, ์์ ์๊ด๊ด๊ณ, ๊ด๊ณ์์
- ๋ฒ๋ธ ์ฐจํธ: ์ฐ์ ๋์ ๊ฐ ์์๋ณ ๋น์ค์ ๋ํจ์ผ๋ก์จ ์ธ๊ฐ์ง ์์ ์๊ด๊ด๊ณ ํฌํ, ๊ฐ๋ก์ถ๋ณ์,์ธ๋ก์ถ๋ณ์,๋ฒ๋ธํฌ๊ธฐ, ์์ ์ง๋ฆ์ ๋ณด๊ณ ํ๋จ
- ํ์คํ ๊ทธ๋จ: ํ๊ท ๊ณผ ์ค์๊ฐ์ ์ฐจ์ด๋ฅผ ๋ช ํํ ์ ์ ์๋ค. ํ๊ท ์ ๊ทน๋จ๊ฐ ์ํฅ๋ฐ์, ์ค์๊ฐ์ ๊ทน๋จ๊ฐ ์ํฅ๋ฐ์ง ์์
- ๋น๊ต ์๊ฐํ
- ํํธ๋งต (Heatmap): ๊ฐ ์นธ์ ์์์ผ๋ก ๋ฐ์ดํฐ ๊ฐ์ ํํ
- ๋ค์ฐจ์์ฒ๋๋ฒ: ์ ์ฌ๋๋ฅผ ๊ธฐ๋ฐ์ผ๋ก ๋ฐ์ดํฐ๋ฅผ ์๊ฐํ
- ๊ณต๊ฐ ์๊ฐํ (Geocoding): ์์น ๊ธฐ๋ฐ ๋ฐ์ดํฐ๋ฅผ ์ง๋์ ์๊ฐํํ์ฌ ํจํด ๋ถ์
์ฃผ์์ฐฝ์ ํํธ๋งต์ด๋ฉด์ ํธ๋ฆฌ๋งต์ด๋ค.
Matplotlib
Matplotlib์ Python์์ ๋ฐ์ดํฐ๋ฅผ ์๊ฐํํ๋๋ฐ ์ฌ์ฉ๋๋ ๊ฐ๋ ฅํ ๋ผ์ด๋ธ๋ฌ๋ฆฌ
๋ฐ์ดํฐ์ ๋ถํฌ, ๊ด๊ณ, ๋ณํ ๋ฑ์ ์ง๊ด์ ์ผ๋ก ๋ณด์ฌ์ฃผ๊ธฐ ์ํด ๋ค์ํ ๊ทธ๋ํ๋ฅผ ์์ฑํ ์ ์๋ค.
๊ทธ๋ํ ์์ฑ ์ ๋ํ์ง์ ์ถ์ ์ค์ ํ์ฌ ๊ทธ๋ํ๋ฅผ ๊ทธ๋ฆด ์ ์๋ ํ๊ฒฝ์ ๋ง๋ จํ๊ณ , plt.plot(), plt.bar(), plt.hist() ๋ฑ์ ํจ์๋ก ๋ฐ์ดํฐ๋ฅผ ์๊ฐํ ํ๋ค.
๋ํ์ง์ ์ถ ๊ฐ์ฒด ์์ฑ
- ๋ํ์ง ๊ฐ์ฒด (figure): ๋ํ์ง ๊ฐ์ฒด๋ ๊ทธ๋ํ๋ฅผ ๊ทธ๋ฆฌ๊ธฐ ์ํ ๊ธฐ๋ณธ ์บ๋ฒ์ค๋ฅผ ์ ๊ณต
- plt.figure(figsize=(x, y)): ๋ํ์ง ํฌ๊ธฐ๋ฅผ ์ค์ . x๋ ๊ฐ๋ก ํฌ๊ธฐ, y๋ ์ธ๋ก ํฌ๊ธฐ
- ์ถ ๊ฐ์ฒด (axes): ์ถ ๊ฐ์ฒด๋ ๋ํ์ง ์์ ํ์๋๋ ๊ทธ๋ํ์ ์ถ์ ์๋ฏธ
- plt.axes(): ๊ธฐ๋ณธ ์ถ ๊ฐ์ฒด ์์ฑ
import matplotlib.pyplot as plt
import numpy as np
# 1) ๋ํ์ง ๊ฐ์ฒด ์์ฑ
plt.figure(figsize=(6, 4))
# 2) ์ ๋ชฉ ์ถ๊ฐ
plt.title('ํ
์คํธ: ํ๊ธ ํฐํธ ํ์ธ')
# 3) ๊ทธ๋ํ ๊ทธ๋ฆฌ๊ธฐ
plt.plot([1, 2, 3], [4, 5, 6])
# 4) ๊ทธ๋ํ ์ถ๋ ฅ
plt.show()
๊ทธ๋ํ์ ์ถ ์ ํ ์ค์
- xlim(): x์ถ์ ๋ฒ์๋ฅผ ์ค์
- ylim(): y์ถ์ ๋ฒ์๋ฅผ ์ค์
plt.figure(figsize=(6, 4))
x = [0, 2, 4, 6, 8]
y = [1, 2, 3, 4, 5]
plt.plot(x, y)
# x์ถ๊ณผ y์ถ์ ๋ฒ์ ์ ํ
plt.xlim(-5, 5) # x์ถ limit ์ข ์ฐ
plt.ylim(-5, 50) # y์ถ limit ์ํ
plt.show()
xlim(-5, 5)์ ylim(-5, 50)์ผ๋ก ์ถ์ ๋ฒ์๋ฅผ ์ ํํ์ฌ, ์ค์ ๋ ์์ญ ๋ฐ์ ๋ฐ์ดํฐ๋ ๋ณด์ด์ง ์์
๋ ์ด๋ธ ๋ฐ ๋ฒ๋ก ์ค์
- plt.xlabel()๊ณผ plt.ylabel(): ๊ฐ๊ฐ x์ถ๊ณผ y์ถ์ ๋ ์ด๋ธ์ ์ถ๊ฐ
- plt.title(): ๊ทธ๋ํ์ ์ ๋ชฉ์ ์ถ๊ฐ
- plt.legend(): ๋ฒ๋ก๋ฅผ ์ถ๊ฐ
plt.plot(np.random.randn(30).cumsum(), 'g-.x', label='2023๋
')
plt.plot(np.random.randn(30).cumsum(), 'b-.x', label='2024๋
')
# ์ถ ๋ ์ด๋ธ๊ณผ ์ ๋ชฉ ์ค์
plt.title('3์ ๋งค์ถ')
plt.xlabel('์๊ฐ(๋จ์: ์ผ)')
plt.ylabel('๋งค์ถ(๋จ์: ์ต์)')
# ๋ฒ๋ก ์ค์
plt.legend(loc='upper right', frameon=False)
# ๊ทธ๋ํ ์ถ๋ ฅ
plt.show()
- label='2023๋ '๊ณผ label='2024๋ '์ ์ฌ์ฉํด ๋ฒ๋ก๋ฅผ ์ถ๊ฐ, plt.legend(loc='upper right')๋ก ๋ฒ๋ก์ ์์น๋ฅผ ์ค์
- frameon=False๋ก ๋ฒ๋ก์ ๋ฐฐ๊ฒฝ ์ญ์
์ด๋ฅผ ํ ๋๋ก
Bar plots, histograms, scatter plots, stack plots, pie charts, fill_between and alpah,
3d scatter plots, subplotting using subplot2grid ๋ฑ์ ์ธ ์ ์๋ค.
๋ค ๋น์ทํ๋ค.
(1) Bar plot (๋ง๋๊ทธ๋ํ)
x = ['A', 'B', 'C', 'D']
y = [3, 5, 2, 4]
plt.bar(x, y)
plt.show()
(2) Histogram (ํ์คํ ๊ทธ๋จ)
data = np.random.randn(1000)
plt.hist(data, bins=30, alpha=0.7, color='blue')
plt.show()
(3) Scatter plot (์ฐ์ ๋)
x = np.random.randn(100)
y = np.random.randn(100)
plt.scatter(x, y, alpha=0.5)
plt.show()
(4) Stack plot (๋์ ๊ทธ๋ํ)
x = np.arange(1, 6)
y1 = [1, 2, 3, 4, 5]
y2 = [2, 3, 4, 5, 6]
y3 = [3, 4, 5, 6, 7]
plt.stackplot(x, y1, y2, y3, labels=['A', 'B', 'C'], alpha=0.7)
plt.legend(loc='upper left')
plt.show()
(5) Pie chart (์ํ ์ฐจํธ)
labels = ['A', 'B', 'C', 'D']
sizes = [15, 30, 45, 10]
plt.pie(sizes, labels=labels, autopct='%1.1f%%')
plt.show()
(6) Fill_between (์์ญ ์ฑ์ฐ๊ธฐ)
x = np.linspace(0, 10, 100)
y = np.sin(x)
plt.fill_between(x, y, alpha=0.5)
plt.show()
(7) 3D Scatter plot
from mpl_toolkits.mplot3d import Axes3D
# 3D ๋ํ์ง ์์ฑ
fig = plt.figure()
ax = fig.add_subplot(111, projection='3d')
# ๋๋ค ๋ฐ์ดํฐ ์์ฑ
x = np.random.rand(100)
y = np.random.rand(100)
z = np.random.rand(100)
# 3D ์ฐ์ ๋
ax.scatter(x, y, z)
plt.show()
(8) Subplot2Grid ์ ์ฌ์ฉํ ์๋ธํ๋กฏ ์์ฑ -> ๋ณต์กํ ๊ทธ๋ฆฌ๋ ๋ ์ด์์ ์์ฑ
fig = plt.figure(figsize=(8, 6))
# ์ ์ฒด 5x2 ๊ทธ๋ฆฌ๋์์ (0, 0)๋ถํฐ ์์, 1ํ 2์ด
ax1 = plt.subplot2grid((5, 2), (0, 0), rowspan=1, colspan=2)
# ๊ทธ๋ํ ์ถ๊ฐ
ax1.plot([1, 2, 3], [4, 5, 6])
ax1.set_title('์๋ธํ๋กฏ ์์')
plt.show()
Seaborn
Matplotlib์ ๊ธฐ๋ฐ์ผ๋ก ๋์ํ๋ฉฐ, ๋ค์ํ ์๊ฐํ ๊ธฐ๋ฅ์ ์ถ๊ฐํ์ฌ ํต๊ณ์ ์ฐจํธ๋ฅผ ์ฝ๊ฒ ๊ทธ๋ฆด ์ ์๋๋ก ๋์์ฃผ๋ ํ์ด์ฌ ๋ผ์ด๋ธ๋ฌ๋ฆฌ
Matplotlib์ ์ฌ์ฉํ ๋๋ณด๋ค ๋ ์ง๊ด์ ์ด๊ณ , ๋ค์ํ ์์ ํ ๋ง์ ์คํ์ผ์ ์ ๊ณต
๊ณต์์ฌ์ดํธ: https://seaborn.pydata.org/
๊ณตํต ํจ์
plt.savefig()
# ๋ชจ๋ Seaborn ํ๋กฏ์ Matplotlib ๊ธฐ๋ฅ์ ์ฌ์ฉํ์ฌ ํ์ผ๋ก ์ ์ฅ ๊ฐ๋ฅ
# ex) plt.savefig('figure.png')
plt.show()
# ํ๋กฏ์ ํ๋ฉด์ ํ์
(1) lmplot (Logistic Model Plot):์ ํ ํ๊ท(๋๋ ๋ก์ง์คํฑ ํ๊ท) ๋ชจ๋ธ์ ์๊ฐํํ๋ ํจ์
- hue ์ธ์๋ฅผ ์ฌ์ฉํ์ฌ ๊ฐ ๋ฒ์ฃผ๋ฅผ ์์์ผ๋ก ๊ตฌ๋ถ
import seaborn as sns
import matplotlib.pyplot as plt
# ๋ฐ์ดํฐ ๋ถ๋ฌ์ค๊ธฐ
sns.set(style="whitegrid")
df = sns.load_dataset('tips')
# lmplot ์์
sns.lmplot(data=df, x='total_bill', y='tip', hue='sex', col='time', palette='muted')
plt.show()
(2)Barplot
sns.barplot(x='day', y='total_bill', data=df, palette='Blues')
plt.show()
(3)์๋ธํ๋กฏ (FacetGrid / FacetPlot)
g = sns.FacetGrid(df, col="sex", row="time")
g.map(sns.scatterplot, "total_bill", "tip")
plt.show()
(4) Despine (์ถ/ํ
๋๋ฆฌ ์ ๊ฑฐ)
sns.set(style="whitegrid")
sns.barplot(x='day', y='total_bill', data=df)
sns.despine()
plt.show()
(5) Lineplot
sns.lineplot(x="size", y="tip", data=df)
plt.show()
(6) ScatterPlot
sns.scatterplot(x="total_bill", y="tip", data=df, hue="time", style="sex")
plt.show()
(7) CountPlot
sns.countplot(x='day', data=df)
plt.show()
(8) Distplot
sns.distplot(df['total_bill'], kde=True, bins=30)
plt.show()
(9) Kdeplot: ์ปค๋ ๋ฐ๋ ์ถ์ (KDE)์ ํตํด ๋ฐ์ดํฐ์ ๋ฐ๋๋ฅผ ์๊ฐํ
sns.kdeplot(df['total_bill'], shade=True)
plt.show()
(10) Boxplot: ๋ฐ์ดํฐ์ ๋ถํฌ์ ์ด์์น๋ฅผ ์๊ฐํํ๋ ์์ ๊ทธ๋ฆผ
sns.boxplot(x='day', y='total_bill', data=df)
plt.show()
(11) Violin Plot
sns.violinplot(x='day', y='total_bill', data=df)
plt.show()
(12) Heatmap
correlation = df.corr()
sns.heatmap(correlation, annot=True, cmap='coolwarm')
plt.show()
(13) PairPlot: ์ฌ๋ฌ ๋ณ์ ๊ฐ์ ๊ด๊ณ๋ฅผ ํ ๋ฒ์ ์๊ฐํํ ์ ์๋ ํจ์
- ๊ฐ ๋ณ์ ๊ฐ์ ์ฐ์ ๋์ ํ์คํ ๊ทธ๋จ์ ๊ทธ๋ฆผ
sns.pairplot(df, hue="sex")
plt.show()
Tuesday (01.14)
์ค๋์ ์ ์ฌ
๋ณ์ โญ๏ธ โญ๏ธ โญ๏ธ โญ๏ธ
์ก์ ๊ตญ๋ฐฅ 10000์...
๋ง์๋ค! ์ก์ ์์ฒด๊ฐ ๋น์ผ๋ฐ, ๋์์๋ ์ก์ ๋จน๊ณ ์ถ์ผ๋ฉด ๋จน์ผ๋ฉด ๋ฑ์ด๋ค!
๋ค์ด๋ฒ ์ง๋
์ก์ ๊ตญ๋ฐฅ ์์๋๋ฆผํ์์
map.naver.com
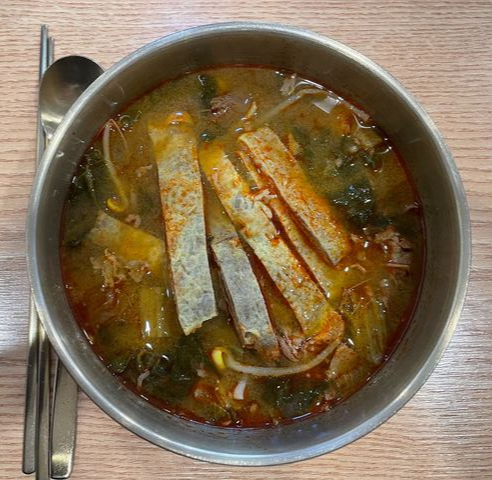
ํ๋ค์ค์์ ์ด ์ด๋ฆ ๋ณ๊ฒฝํ๋ ๋ฒ
https://docs.kanaries.net/ko/topics/Pandas/pandas-rename-column
Plotly
- ์ธํฐ๋ ํฐ๋ธ ๊ทธ๋ํ ์์ฑ
- dictํ์์ผ๋ก ๋ช ๋ น์ด ์์ฑ
- JSON ํ์์ผ๋ก ์ ์ฅ
- ๋ค์ํ๋ฐฉ์์ผ๋ก Export
px.data.tips() # Plotly Express์์ ์ ๊ณตํ๋ ๋ด์ฅ ๋ฐ์ดํฐ์
๋ถ๋ฌ์ค๋ ํจ์
# include='all'์ ์ฌ์ฉํ์ฌ ์์นํ ๋ฐ์ดํฐ๋ฟ๋ง ์๋๋ผ ๋ฒ์ฃผํ ๋ฐ์ดํฐ์ ๋ํ ์์ฝ ํต๊ณ๋ ์ถ๋ ฅ
tips.describe(include='all')
tips.info()
tips.day.unique() # day ์ปฌ๋ผ์ ๊ณ ์ ํ(unique) ๊ฐ๋ค์ ๋ฐํ
tips['size'] # tips ๋ฐ์ดํฐํ๋ ์์์ size ์ปฌ๋ผ ์ ํ
gapminder = px.data.gapminder()
# ์ฐ์ ๋ ์์ฑ
fig = px.scatter(
gapminder, # ๋ฐ์ดํฐ ์ด๋ฆ (๋ฐ์ดํฐํ๋ ์ ํ์์ด์ด์ผ ํจ)
x='gdpPercap', # x์ถ์ ํ์ํ ๋ฐ์ดํฐ ์ปฌ๋ผ (1์ธ๋น GDP)
y='lifeExp', # y์ถ์ ํ์ํ ๋ฐ์ดํฐ ์ปฌ๋ผ (ํ๊ท ๊ธฐ๋ ์๋ช
)
color='continent', # ๋๋ฅ๋ณ ์์ ๊ตฌ๋ถ
size='pop', # ์์ ํฌ๊ธฐ๋ก ์ธ๊ตฌ๋ฅผ ํ์
hover_name='country', # ๋ง์ฐ์ค ์ค๋ฒ ์ ํ์๋ ์ต์๋จ ์ ๋ณด (๊ตญ๊ฐ ์ด๋ฆ)
log_x=True, # x์ถ ๋ก๊ทธ ์ค์ผ์ผ ์ ์ฉ
title='Life Expectancy vs GDP Per Capita - Scatter Plot', # ๊ทธ๋ํ ์ ๋ชฉ
labels={'gdpPercap': 'GDP Per Capita', 'lifeExp': 'Life Expectancy'}, # ์ถ ๋ ์ด๋ธ ๋ณ๊ฒฝ
width=800, # ๊ทธ๋ํ์ ๊ฐ๋ก ํฌ๊ธฐ
height=600 # ๊ทธ๋ํ์ ์ธ๋ก ํฌ๊ธฐ
)
# ๊ทธ๋ํ ์ถ๋ ฅ
fig.show()
์ด๋ฒ์๋ iris ๋ฐ์ดํฐ
px.data.iris()
iris.describe(include='all') # species_id: ์ง์ ๋ณ์(๋ฌธ์์ด, ๋ฒ์ฃผํ - setosa, versicolor, virginica)
gapminder.describe(include='all').T # ํ์ด ์ ์น
iris.columns #์ปฌ๋ผ ํ์ธ
# ์ปฌ๋ผ๋ช
๋ณ๊ฒฝ
# inplace=True: ์๋ณธ ๋ฐ์ดํฐํ๋ ์์ ์ง์ ์์ -> ์๋ก์ด ๋ฐ์ดํฐํ๋ ์์ ์์ฑํ์ง ์๊ณ ๊ธฐ์กด ๊ฐ์ฒด ์์ฒด๊ฐ ๋ณํจ
iris.rename(columns={'sepal_length':'๊ฝ๋ฐ์นจ๊ธธ์ด', 'sepal_width':'๊ฝ๋ฐ์นจ๋๋น','petal_length':'๊ฝ์๊ธธ์ด','petal_width':'๊ฝ์๋๋น'},inplace=True)
1) Scatter Plot
facet_row: ์ง์ ์ปฌ๋ผ ๊ธฐ์ค์ผ๋ก subplot์ ๊ทธ๋ฆผ
fig = px.scatter(tips, x='total_bill', y='tip', color='sex', facet_row='day')
from sklearn.datasets import load_iris
iris = load_iris()
print(iris.DESCR) # ์์ด๋ฆฌ์ค ๋ฐ์ดํฐ์
์ ๋ํ ์ค๋ช
์ ํฌํจํ๋ ์์ฑ
# ๋๋ฅ๋ณ๋ก ์๊น์ ์ ํจ
px.scatter(gap2007, x='gdpPercap', y='lifeExp', color='continent')
#x์ถ์ ๋ก๊ทธ ์ค์ผ์ผ, ๋ฐ์ดํฐ๋ฅผ ๊ธฐ๋ฐ์ผ๋ก ํ๊ท์ ์ถ๊ฐ lowess: ๋ก์ปฌ ํ๊ท์
px.scatter(gap2007,log_x=True, title='2007๋
์ ๋๋ฅ๋ณ ๊ธฐ๋์๋ช
', trendline='lowess')
2) ์ฐ์ ๋ ๋งคํธ๋ฆญ์ค(Scatter Matrix): ์ฌ๋ฌ ๋ณ์ ๊ฐ์ ๊ด๊ณ๋ฅผ ํ ๋ฒ์ ๋ณด๊ธฐ ์ํด ์ฐ์ ๋ ๋งคํธ๋ฆญ์ค๋ฅผ ์์ฑํ๋ ํจ์
๊ฐ ๋ณ์ ๊ฐ์ scatter plot๊ณผ ๋๊ฐ์ ์์ ํ์คํ ๊ทธ๋จ์ ํจ๊ป ๊ทธ๋ ค์ค
# px.scatter_matrix๋ seaborn์ pairplot๊ณผ ๋งค์ฐ ์ ์ฌํ ๊ธฐ๋ฅ์ ์ํ
px.scatter_matrix(gap2007): gap2007์ ๋ชจ๋ ๋ณ์๋ฅผ ๊ธฐ์ค์ผ๋ก ์ฐ์ ๋ ๋งคํธ๋ฆญ์ค๋ฅผ ๊ทธ๋ฆฐ๋ค
px.scatter_matrix(gap2007, ['year', 'pop', 'lifeExp', 'gdpPercap']): ์ธ์๋ฅผ ์ฌ์ฉํ์ฌ ์ฐ์ ๋ ๋งคํธ๋ฆญ์ค๋ฅผ ๊ทธ๋ฆด ๋ณ์๋ค์ ์ง์
3) Line Chart
๊ฐ ์์์ ๊ตญ๊ฐ์ ๊ธฐ๋์๋ช ์ด ์๊ฐ์ด ํ๋ฆ์ ๋ฐ๋ผ ์ด๋ป๊ฒ ๋ณํํ๋์ง๋ฅผ ๋ณด์ฌ์ฃผ๋ ๋ผ์ธ ์ฐจํธ
px.line(gapAsia, x='year', y='lifeExp', color='country')
4) ์๊ณ์ด ๊ทธ๋ํ
<์ ์ฒด๊ธฐ๊ฐ>
import plotly.express as px
import yfinance as yf
# ์ผ์ฑ์ ์์ ์ฃผ์ ๋ฐ์ดํฐ ๋ค์ด๋ก๋
samsung = yf.download('005930.KS', start='2020-01-13', end='2025-01-13')
# ๋ฐ์ดํฐ ๋ฆฌ์
๋ฐ ์ปฌ๋ผ ์ด๋ฆ ์กฐ์
samsung = samsung.reset_index()
# ์ปฌ๋ผ์ ๋ฉํฐ ์ธ๋ฑ์ค๋ฅผ 1์ฐจ์์ผ๋ก ๋ณ๊ฒฝ: ์ปฌ๋ผ ์ด๋ฆ์ด ๋ฉํฐ ์ธ๋ฑ์ค(pandas์์ ํ ์ด์ ๋๊ฐ ์ด์ ์ธ๋ฑ์ค) ํํ๋ก ๋์ด ์์ ๋,
๋ ๋ฒ์งธ ๋ ๋ฒจ์ ์ธ๋ฑ์ค๋ฅผ ์ ๊ฑฐํ์ฌ ์ปฌ๋ผ ์ด๋ฆ์ 1์ฐจ์์ผ๋ก ๋ง๋๋ ์์
samsung.columns = samsung.columns.droplevel(1)
# ์ ์ฒด ๊ธฐ๊ฐ์ ๋ํ ์๊ณ์ด ๊ทธ๋ํ
fig = px.line(samsung, x='Date', y='Close', title='Samsung Stock Closing Price Over Time')
fig.show()
<ํน์ ๊ธฐ๊ฐ: range_x>
# ํน์ ๊ธฐ๊ฐ(2022๋
12์ 31์ผ๋ถํฐ 2024๋
1์ 31์ผ๊น์ง)์ ์๊ณ์ด ๊ทธ๋ํ
fig2 = px.line(samsung, x='Date', y='Close', range_x=['2022-12-31', '2024-01-31'], title='Samsung Stock Closing Price from Dec 2022 to Jan 2024')
fig2.show()
streamlit์ ์ฌ์ฉํด๋ณด์
- ๊ฐ์ํ๊ฒฝ(ํ์ด์ฌ) -> ์ธ์ถ์ฉ ๊ฐ๋ฐฉ, ์ฌํ์ฉ ๊ฐ๋ฐฉ ์ฒ๋ผ ๋ชจ๋ ๊ฑธ ์ธ๋ค๊ณ ๊ฐ๋๊ฒ ์๋๋ผ, ๋ชฉ์ ์ ๋ง๋ ํจํค์ง๋ค๋ง ๋ฐ๋ก ๋ง๋ค์ด๋์ ์์์ ๊ฐ์ํ๊ฒฝ.
- ์ด๋ฅผ ํ์ด์ฌ ์์์ ๋๋ฆผ
import streamlit as st
# text๋ฅผ ์
๋ ฅํ๋ ๊ฒ์์ฐฝ ํ๋ ๋ง๋ค๊ธฐ
# anly_list์ ์๋ ๊ธ์๊ฐ ์ผ๋ถ๋ผ๋ ๋ค์ด๊ฐ๋ฉด
# ์ด๋ฏธ์ง ๋ฆฌ์คํธ์ ์๋ ๊ฒ์์ฐฝ์ ํ๋ ๋ง๋ค์ด์ฃผ์ธ์
word= st.text_input("๊ฒ์ํ์ค ์ ๋๋ฉ์ด์
์ ์
๋ ฅํ์ธ์")
ani_list = ['์งฑ๊ตฌ๋๋ชป๋ง๋ ค', '๋ชฌ์คํฐ','๋ฆญ์ค๋ชจํฐ']
img_list = ['https://i.imgur.com/t2ewhfH.png',
'https://i.imgur.com/ECROFMC.png',
'https://i.imgur.com/MDKQoDc.jpg']
dict={}
for i in range(len(ani_list)):
dict[ani_list[i]] = img_list[i]
if not word:
st.text("No title")
else:
for ani in ani_list:
if word in ani:
st.image(dict[ani], width=1000)
Wednesday(01.15)
์ค๋์ ์ ์ฌ
๋ณ์ โญ๏ธ โญ๏ธ โญ๏ธ
๋์ง๋ฎ๋ฐฅ์ ๋จน์๋ค.
์ข ํฉ๋ถ์์ ์น๊ณ ๋ ๊ด์ฐฎ์ ๋ง์ด์๋๋ฐ, ๊ฐ์ด ๋จน์ ๋จํด์์ ์จ ์น๊ตฌ๋ ๋์ง์ ์ ์ ํจ์ด ๋ถ์กฑํ๋ค๊ณ ํ๋ค.
์ญ์ ๋ฐ๋ท๊ฐ์์ ์จ ์น๊ตฌ๋ผ ํด์ฐ๋ฌผ์ ๋ํ ๊ธฐ์ค์ด ๋์๊ฒ ๊ฐ๋ค.
๋ฌผ๊ณ ๊ธฐ ๋จน๊ณ ์ถ์ผ๋ฉด ์ฐฝ๋ฌธ์์ ๋์ ํด์ ๋จน์ ์ ์๋ค๋ผ๋ ๋ง์ด ...
๋ค์ด๋ฒ ์ง๋
์ต๊ฐ๋ค๊น๋ฐฅ
map.naver.com
Thursday(01.16)
์ค๋์ ์ ์ฌ
๋ณ์ โญ๏ธ โญ๏ธ โญ๏ธ โญ๏ธ
๋ฏธ๋ถ๋น์ ์ด๋ ์ง์ ์ ๊ฐ๋ ํญ์ ์ค๊ฐ ์ด์์ ํ๋ ๊ฒ ๊ฐ๋ค.
๊ณ ๊ธฐ๊ฐ ์ ๋ง ๋๋ํ๋ค ใ ใ
๋ค์ด๋ฒ ์ง๋
๋ฏธ๋ถ๋น ์์DMC์
map.naver.com
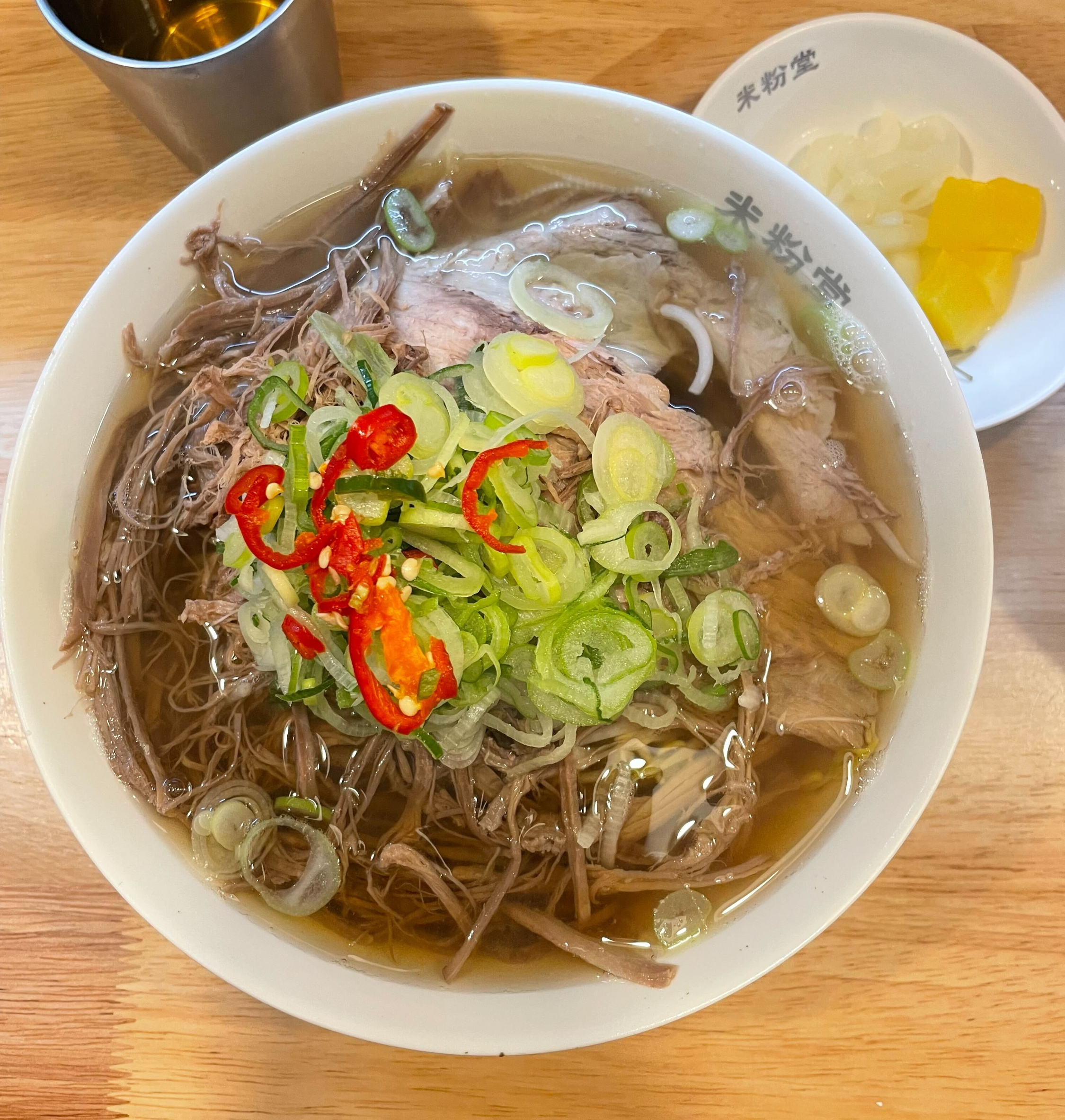
ํํ๋ก์ ํธ ์งํ
์ด๋ฒ์ฃผ ์์์ผ, ๋ชฉ์์ผ์ ํฉ์ณ์ ๊ธฐ๋กํ๊ฒ ๋ค. ์ดํ๋์ ๊ฐ๋จํ ํ ํ๋ก์ ํธ๋ฅผ ์งํํ์๋ค. (์์์ผ ์คํ, ๋ชฉ์์ผ ์ค์ )
์ด๋ฒ์ฃผ์ ๋ฐฐ์ด ๋ฐ์ดํฐ ์๊ฐํ ํด๊ณผ steramlit์ ์ด์ฉํ์ฌ, ํ๋์ ์ฌ์ดํธ๋ฅผ ๋ง๋๋ ์์ ์ด์๋ค.
5๋ช ์ด์ ์์ ํ์๊ณ , ๊ฐ๋ฐ์๋ 8์๊ฐ์ ๋์ ์๊ฐ๋ฐ์ ์์ด์ ๊ธํ๊ฒ ๋ง๋ค์๋ค.
์ฃผ์ ์ ๋ํ ํฌ์ธํธ๋ฅผ FISA ์๊ฐ์๋ค์๊ฒ ์ฌ์ฉํ๊ธฐ ์ ํฉํ ์ฃผ์ ๋ก ์๊ฐํ๋ค๊ฐ ๋์จ ๊ฒ์ด ์๋น์ ๋ณด๋ฅผ ์ ์ฅํ๋ ํ๋ก์ ํธ์๋ค.
๋ฌผ๋ก , ์นด์นด์ค๋งต์ด๋ ๋ค์ด๋ฒ ์ง๋์๊ฐ์ ๋ ์ข์ ์ฑ์ด ์กด์ฌํ์ง๋ง, ์๊ฐ์๋ค์ ํ๊ฒ์ธต์ผ๋ก ์๊ฐํด๋ดค์ ๋๋, ์ ์ฒด ๊ฑฐ๋ฆฌ๊ฐ ์๋ ์์๋ ๊ทผ์ฒ ์๋น ์์ฃผ๋ก ๋ง์ถคํ์ผ๋ก ๋ณด์ฌ์ฃผ๋๊ฒ์ด ์ข๋ค๊ณ ํ๋จํ์๋ค.
ํญ์ 1์๊ฐ์ ์ ์ฌ์๊ฐ์, ๋ฐฅ์๋จน๊ณ ๋์์์ผํ๋๋ฐ, ์กฐ๊ธ ๋จผ ๊ฐ๊ฒ๋ฅผ ๊ฐ ๊ฒฝ์ฐ ์๊ฐ์์ ๋์ฐฉํ๊ธฐ ์ ๋งคํ ๋๊ฐ ์์ด์, ์ด ์ฌ์ดํธ๋ฅผ ์ฐธ๊ณ ํ๋ค๋ฉด ์ข์ ๋๊ตฌ๋ก ์ธ ์ ์์๊ฒ ๊ฐ์๋ค.
๋ฐ๋ณ๋ก ์ด๋ค ์์์ ์ ๋ง์ด๊ฐ๊ณ , ๊ต์ก์ฅ์์์ ๊ฐ๊ฒ๊น์ง ์ผ๋ง๋ ๊ฑธ๋ฆฌ๊ณ , ๋ค๋ฅธ ์๊ฐ์๋ค์ ์ด๋๋ฅผ ๊ฐ๋ค์๋์ง๋ฅผ ํ๋ฒ์ ๋ณผ ์ ์๋ ๋ง์ถคํ ์ฌ์ดํธ๋ฅผ ๋ง๋ค๊ฒ ๋์๋ค.
- ํผ ํ์์ผ๋ก ๋จน๊ณ ์จ ์ ์ฌ์ ๊ธฐ๋กํ๋ ์ฐฝ
- ๋ฐ๋ณ, ์ฃผ์ฐจ๋ณ ์ด๋ค ์๋น์ ๋ง์ด ๊ฐ๋์ง ํ์ธํ๋ ์ฐฝ
- ๊ต์ก์ฅ์์ ๊ฐ๊ฒ์ ์์น๋ฅผ ํ์ธํ๋ ์ฐฝ
- ๋ค๋ฅธ ์๊ฐ์๋ค์ด ๊ฐ๋ค์จ ํ๊ธฐ๋ฅผ ํ์ธํ๋ ์ฐฝ
์ด๋ ๊ฒ 4๊ฐ์ง๋ก ๊ตฌ์ฑ๋๋ค.
์ด๋ฒ์ ์์ ์์ ๋ฐฐ์ด ๋์๋ณด๋ ๋ง๋๋ ๋ถ๋ถ์ ํด๋ณด๊ณ ์ถ์๋๋ฐ, ๋ค๋ฅธ ๋ถ๋ถ์ ๋งก์์ ํด์ผํ ๊ฒ ๊ฐ์ ํ์๋ค์๊ฒ ๋ถํํ๊ฒ ๋์๋ค.
๋ด๊ฐ ๋งก์ ๋ถ๋ถ์ DB์ฐ๊ฒฐ ๋ฐ ๊ตฌ์ถ, ์ฌ์ง ์ ์ฅ์ ๋ํ ๋ถ๋ถ, ์ง๋, ํ๊ธฐ์ ๋ํ ๊ฒ์๊ธ ์ด๋ ๊ฒ ๋งก์๋ค.
๊ฐ ๋ถ๋ถ์ ๊ฐ๋จํ ์ค๋ช ํ๋ฉด
- ์กด์ฌํ๋ ๋ฐ์ดํฐ๊ฐ ์๋ ์๊ฐ์๋ค์ ์ ๋ณด๋ฅผ ๋ฐ์์ ํด์ผํ๊ธฐ์ DB ์ฌ์ฉ์ ํ์ ์๊ธฐ์, ์ ๋ณด ์ ์ฅ๊ณผ ๋ถ๋ฌ์ค๋ ์์
- ์ฌ์ง์ ๊ฒฝ์ฐ S3์ ์ผ๋ฐ์ ์ผ๋ก ์ ์ฅํ๋๋ฐ, ํด๋ผ์ฐ๋ ์ฐ๊ฒฐ์ ์์ด ํธ๋ฌ๋ธ ์ํ ์ด ์๊ธธ๊ฑฐ๋ผ ํ๋จํ์ฌ, ์ฌ์ง ์์ฒด๋ฅผ BLOB์ผ๋ก ์ ์ฅํ์๋ค. ํ ์์ ์์ 100๊ฐ ์ ๋์ ๋ฐ์ดํฐ์ด๊ธฐ์, DB ์ ๊ทผ ์๊ฐ์์๋ ํฐ ๋ฌธ์ ๊ฐ ์์๊ฑฐ๋ผ ํ๋จํ์๋ค.
- ์ง๋๋ OSM์ ์ฌ์ฉํ๋ค. ๋ฌด๋ฃ์ธ ๋ถ๋ถ๋ ์์๊ณ , (๋ฌผ๋ก ์นด์นด์ค๋ ์ด์ ๋ API ์์ฒญ์ ๋ฌด๋ฃ๋ค.) ์นด์นด์ค๋ ๋ค์ด๋ฒ์ง๋์ ๊ฒฝ์ฐ ์ถ๊ฐ์ ์ผ๋ก ๊ฐ์ ธ์ค๋ ์ ๋ณด๊ฐ ๋ง๋ค๊ณ ๋๊ปด์ ์ด๋ฅผ ์ฌ์ฉํ๊ณ , ๋ง์ปค๋ Folium ๋ผ์ด๋ธ๋ฌ๋ฆฌ๋ฅผ ์ฌ์ฉํ์ฌ ์ง๋์ ํ์ํ์๋ค.
- DB์ ์ ์ฅ๋ ์ฌ์ฉ์ ํ๊ธฐ๋ฅผ ํ ์คํธ, ์ฌ์ง์ผ๋ก ๋ถ๋ฌ์์ ๋ณด์ฌ์ฃผ๊ณ , ํ์ด์ง๋ค์ด์ ๊ณผ ์ค๋ฅ์ฒ๋ฆฌ๋ฅผ ํ์๋ค.
๋ชฉ์์ผ์ ๋ฐํ๋ฅผ ํ๊ฒ ๋์๊ณ , ๋ฐํ์ง์ ๊น์ง ์๊ฐ์ด ๋ถ์กฑํ๋ ๋ถ๋ถ์ 2๊ฐ์ง์๋ค.
ํธ๋ฌ๋ธ ์ํ
๋ด๊ฐ ๊ฒช์ ํธ๋ฌ๋ธ ์ํ ๋ง ๊ธฐ๋กํ์๋ฉด,
1. ์ง๋๋ฅผ ๋งคํํด์ผ ํ๋๋ฐ, '๊น์น๋๊ฐ'๋ผ๋ ์๋น์ด ์์ ๋,"๊น์น๋๊ฐ ์์"์ ๋์ค๊ณ , "๊น์น๋๊ฐ ์์์ "์ ์ ๋์ค๋ ๋ฌธ์
OSM์ ๋ถ์กฑํ ๋ถ๋ถ์ธ๊ฒ ๊ฐ๋ค.
2. ์ ์ฑ์ ์ ๋ ๊ฐ๋ค์ค์ง ์์ ์ฅ์๋ฅผ ๊ฐ๋ค์๋ค๊ณ ํ ๊ฒ + ์ค์ ๋ก ์กด์ฌํ์ง ์๋ ์ฅ์๋ฅผ ์ ๋ ฅํ ๊ฒฝ์ฐ
๊ธ๋ฐฉ ์ฒ๋ฆฌํ ์ ์์๋๋ฐ, ์ด๊ฒ์กฐ์ฐจ ํ ์๊ฐ์ด ์๋์ด์ ๋ฐํ๋๋๊ณ ์งํ์ ํด๋ณด๊ธฐ๋ก ํ์๋ค.
๋ด๊ฐ ์๊ฐํ ํด๊ฒฐ๋ฐฉ์์ OSM์ ๋ฑ๋ก๋ ๊ฐ๊ฒ์ ๊ฒฝ์ฐ, ๊น์น๋๊ฐ๋ฅผ ์๋ฅผ ๋ค๋ฉด "๊น์น๋๊ฐ ์์", "๊น์น๋๊ฐ ์์์ " ์ด๋ฐ์์ผ๋ก ์ด๋์ ๋์ ํ์์ ๊ณ ์ ๋์ด ์๋ค. ๋ฐ๋ผ์, ์ด๋ฅผ ๋์ ๋๋ฆฌ ํํ๋ก ๋งคํํด์,
{"๊น์น๋๊ฐ" : "๊น์น๋๊ฐ ์์", "๊น์น๋๊ฐ ์์์ " }
์ด๋ฐ ๋ฐฉ๋ฒ์ผ๋ก ํด๊ฒฐํ๋ค๋ฉด, ์ค์ ๋ก ๊ฐ๊ฒ๊ฐ ์๊ฑฐ๋, ์์์ด ์๋๋ผ์ 1์๊ฐ๋ด๋ก ๋จน๊ณ ์ฌ ์ ์๋ ๊ฐ๊ฒ๋ฅผ ์ ๋ ฅํ ๊ฒฝ์ฐ๋ค์ ๋ฐฉ์งํ ์ ์๊ณ , ์ต๋ํ ๋ง์ ๊ฐ๊ฒ๋ฅผ ์ง๋์ ํ์ํ ์ ์์ ๊ฒ ๊ฐ๋ค. ์๊ฐ์ ์ฌ์ ๊ฐ ์์ ๋ ํด๊ฒฐํด ๋ณด๋ ค๊ณ ํ๋ค. 1/23 ๊ธฐ์ค ํด๊ฒฐ์๋ฃ
๋ฐํ๋ ํฐ ๋ฌธ์ ์์ด ์งํํ๊ณ , 5๊ฐ์ ๋์ ์ง๋ฌธ์ ๋ฐ์๋๋ฐ, ์ง๋ฌธ ๋ชจ๋ ๊ฐ์ํ๊ณ ์์๋ ํธ๋ฌ๋ธ ์ํ ์ ๋ํ ๋ถ๋ถ์ด์๋ค.
ํ๋ก์ ํธ๋ฅผ ์ํ ๋ฐ์ดํฐ๋ฅผ ๋ฐ๊ธฐ ์ํด์๋ ์ฌ๋์์ ํ๋ณด๋ฅผ ํด์ผ ํ๊ณ , ๋ฐํ ๋๋๊ณ ์ปคํผ ์ถ์ฒจ์ ํ์๋๋ฐ, ์ฒ์์ ํ์์ด ๋น์ฒจ๋์ด์ ์ข์ํ๋ ๋ชจ์ต์ด ์๊ฒผ๋ค.
ํด๋ํฐ ๋ท๋ฒํธ ๋๋ฆผํ์ ๋ฃ๊ณ ๋๋ฆผํ ๋๋ฆฌ๋๋ฐ, ๋ฒํธ ๋์ค๋๊น 1์ด ์๋ค๊ฐ "์ด! ๋๋ค" ํ๋๊ฒ ์ด์ด๊ฐ ์์ผ๋ฉด์ ์๊ฒผ๋ค. (์ฟ ํฐ์ ๋ค์ ์ถ์ฒจํด์ ๋ค๋ฅธ ์ธ์์๊ฒ ๋์๊ฐ๋ค.)
์ด ์ ๋ ์งง์ ์๊ฐ์ ํ๋ก์ ํธ๋ฅผ ํ ๊ฒฝ์ฐ๊ฐ ์ ์ด์, ์๊ฐ์ ๋ํ ์๋ฐ๊ฐ์ด ์ปธ๋ค. ๊ทธ๋์ ๋ชฉ์์ผ ์ ์ฌ์ ์ ๋ง ๋์ถฉ ๋จน๊ณ ์งํ ํ๊ณ , ๊ทธ๋๋ ๋ง๋ฌด๋ฆฌ๋ฅผ ์ ํด์ผํ๊ธฐ์, ์ฑ ์๊ฐ์ ๊ฐ์ง๊ณ ๋ง๋ฌด๋ฆฌ ํ๋ ๊ฒ ๊ฐ๋ค.
ํ์์ด ๊ณ ์ํ๋ค๊ณ ํด์ค์ ๋ฟ๋ฏํ ํ๋ก์ ํธ์๋ค.
https://github.com/FISAFOODIE/main
https://fisafoodie.streamlit.app/
Friday(01.17)
์ค๋์ ์ ์ฌ
๋ณ์ โญ๏ธ โญ๏ธ โญ๏ธ โญ๏ธ
JTBC ๊ตฌ๋ด์๋น์์ ์์ฌํ๋ค.
6500์์ด๋ผ๋ ๊ฐ๊ฒฉ์ ์ด์ ๋๋ฉด ๊ฑฐ๋ฆฌ๊ฐ ์กฐ๊ธ ๋จผ๊ฑธ ๊ฐ์ํ๋๋ผ๋ ๋จน์๋ง ํ๋ค.
๋ค์ด๋ฒ ์ง๋
์ ์ธ๊ณํธ๋ ์ค์๊ทธ๋ฃน์
map.naver.com
์ค๋์ SQL ์์ฃผ๋ก ๋ฐฐ์ ๋ค. ๊ฐ๋จํ DB์ ๊ดํ ์ ๋ฆฌ๋ง ํ๊ฒ ๋ค.
๋ฐ์ดํฐ๋ฒ ์ด์ค ๊ด๋ จ ์ ๋ฆฌ: https://koreatstm.tistory.com/156
๋ฐ์ดํฐ์ ์ข
๋ฅ
- ์ ํ
- ๋ฐ์ ํ: json, xml, html, yaml
- ๋น์ ํ: ํ ์คํธ, ์ด๋ฏธ์ง
๋น ๋ฐ์ดํฐ: ์ ํ&๋ฐ์ ํ&๋น์ ํ
- ํน์ฑ
- ๋ค์์ฑ
- ๊ท๋ชจ
- ์๋
- ์ถ๊ฐ๋ก ๊ฐ์น, ๋ณ๋์ฑ,์ ํ์ฑ,๋ณต์ก์ฑ
- ๋ฐ์ดํฐ๋ฒ ์ด์ค: ์ฌ๋ฌ ์ฌ๋์ด ์ฌ์ฉ๊ฐ๋ฅํ ๋ฐ์ดํฐ ์งํฉ, ๋ ผ๋ฆฌ์ ์ธ ๊ฐ๋
- DBMS: ๋ฐ์ดํฐ๋ฒ ์ด์ค ๊ด๋ฆฌ ์์คํ
- ๋ฆด๋ ์ด์ : ํ๊ณผ ์ด๋ก ๊ตฌ์ฑ๋ ํ ์ด๋ธ -> ํ ์ด๋ธ๊ณผ ์ ์ฌํ์ง๋ง, ๋ ผ๋ฆฌ์ ์ธ ๊ฐ๋ ์์ '๋ฆด๋ ์ด์ '์ด ๋ ๊ฐ์กฐ๋จ. ํ ์ด๋ธ์ ๋ฌผ๋ฆฌ์ ์ธ ๊ฐ์ฒด์ด๋ฏ๋ก, ํ ์ด๋ธ๊ณผ ๋ฆด๋ ์ด์ ์ ์ฐจ์ด๋ ๋ ผ๋ฆฌ์ /๋ฌผ๋ฆฌ์ ๊ด์ ์ ์ฐจ์ด์์ ๋ฐ์
- ํ ์ด๋ธ: 2์ฐจ์ ํํ์ ๋ฐ์ดํฐ๋ฒ ์ด์ค ๊ฐ์ฒด, ํ ํํ
- ์คํค๋ง: ๋ฐ์ดํฐ๋ฒ ์ด์ค ๊ตฌ์กฐ๋ฅผ ์ ์ํ๋ ๋ ผ๋ฆฌ์ ์ค๊ณ
- MySQL์ ๋ ผ๋ฆฌ์ ์คํค๋ง ๊ณ์ธต๊ณผ ๋ฌผ๋ฆฌ์ ๋ฐ์ดํฐ๋ฒ ์ด์ค ๊ณ์ธต์ ๋ช ํํ์ง ์์์ CREATE DATABASE ์ CREATE SCHEMA๊ฐ ๋์ผํ๊ฒ ๋์ํ์ฌ ํท๊ฐ๋ฆฐ๊ฒ
- ์ธ์คํด์ค: ์คํค๋ง์ ๋ฐ๋ผ ์ค์ ์ ์ฅ๋ ๋ฐ์ดํฐ ์งํฉ
์ดํธ๋ฆฌ๋ทฐํธ=์ด=์์ฑ=ํ๋=์ปฌ๋ผ
๋ก์ฐ=ํ=ํํ=๋ ์ฝ๋
Data Mart & Data Warehouse & Data Lake
- ๋ฐ์ดํฐ ๋งํธ: ๋ฐ์ดํฐ ์จ์ดํ์ฐ์ค์ ํ์ ์งํฉ์ผ๋ก, ๋น์ฆ๋์ค ์ฉ๋๋ฅผ ์ํด ์ ์ ๋ ๋ฐ์ดํฐ ์ ๊ณต
- ๋ฐ์ดํฐ ์จ์ดํ์ฐ์ค: DM์ ๊ณต๊ธํ ๋ฐ์ดํฐ๋ฅผ raw data๋ฅผ ๊ฐ์ง๊ณ ์ฃผ์ ๋ณ๋ก ์ ์ฅํจ์ด๋, ๋ฐ์ดํฐ ์ ์ ํ ๋ ๋ฒ๋ ค์ง๋ ๋ฐ์ดํฐ์ ๋น์ฉ์ด ๋ค์ด์ DL์ด๋ผ๋ ์๋ก์ด ๊ตฌ์กฐ๊ฐ ๋ฐ์
- ๋ฐ์ดํฐ ๋ ์ดํฌ: raw data๋ฅผ ๊ทธ๋๋ก ๊ฐ์ ธ์ ์ ์ฅ, ๋ค์ํ ํ์ ์ง์
DBMS ์ ํ
์ฌ์ฉ์๊ฐ DB์ ๋ํ ์์ธ์ค๋ฅผ ์ ์, ์์ฑ, ์ ์ง, ์ ์ด ํ ์ ์๋๋ก ์ํํธ์จ์ด์์ฉํ๋ก๊ทธ๋จ
- ๊ณ์ธต์ DBMS: ํธ๋ฆฌ์ ๊ฐ์ ๊ตฌ์กฐ → ์ผ๋๋ค ๊ด๊ณ์ ํจ์จ์ ์ด์ง๋ง ์ ์ฐ์ฑ์ด ๋จ์ด์ง๋ค.(๋ณ๊ฒฝ ๊น๋ค๋กญ๊ณ ์ํ๋ ์ ๋ณด ์ฐพ๊ธฐ ํ๋ค๋ค), ํ์ฌ๋ ์ฌ์ฉ ์ํจ
- ๋คํธ์ํฌ DBMS: ๋ค๋๋ค ์ง์, ๊ณ์ธต์ DBMS๋ณด๋ค๋ ์ ์ฐํ์ง๋ง, ์ค๊ณ,๊ด๋ฆฌ๊ฐ ๋ณต์ก
- RDBMS: ํ ์ด๋ธ ๋จ์(ํ&์ด)๋ก ๊ตฌ์ฑ๋จ, SQL๋ก ์กฐ์, ์ฌ๋ฌ ์ ์ฝ์กฐ๊ฑด์ผ๋ก ๋ฌด๊ฒฐ์ฑ ์กด์ฌ
- NoSQL(Not Only SQL): ํ์ฅ์ฑ, ๋ถ์ฐ ์ ์ฅ, ๋น(๋ฐ)์ ํ ๋ฐ์ดํฐ ์ฒ๋ฆฌ์ ์ ํฉ, Key Value(Redis), Document(Mongo), Graph(Neo4j), Column Family(Casandra) ์ข ๋ฅ๋ก ๊ตฌ๋ถ๋๋ฉฐ, ๋น ๋ฐ์ดํฐ์ ์ ํฉํ๋ค.
๋ฐ์ดํฐ ์ธ์ด
https://koreatstm.tistory.com/157#3.%20%EB%8D%B0%EC%9D%B4%ED%84%B0%20%EC%96%B8%EC%96%B4-1
SQL ์ฐ์ต์ ํ๋ก๊ทธ๋๋จธ์ค์์ ํ๊ธฐ ์ข๋ค. ์ด์ ์ SQLD ์ค๋นํ ๋ ํ์์๋๋ฐ, ์์ง ๋ชป ํผ ๋ฌธ์ ๋ค์ด ์๋ค.
https://school.programmers.co.kr/learn/challenges?tab=sql_practice_kit
์ด๋ฒ์ฃผ SQL ์์ ํ๋ ๊น์, ๋จ์ 20๋ฌธ์ ๋ฅผ ๋ค ํ์ด๋ด์ผ๊ฒ ๋ค.
์ฝ๋๋ ๋ฐ๋ก ๊ณต์ ํ๊ฒ ๋ค.
'๐ฐ Woori FISA > ํ๊ธฐ' ์นดํ ๊ณ ๋ฆฌ์ ๋ค๋ฅธ ๊ธ
์ฐ๋ฆฌ FISA AI์์ง๋์ด๋ง 6์ฃผ์ฐจ ๊ณผ์ (0) | 2025.02.11 |
---|---|
์ฐ๋ฆฌ FISA AI์์ง๋์ด๋ง 5์ฃผ์ฐจ ๊ณผ์ (0) | 2025.02.03 |
์ฐ๋ฆฌ FISA AI์์ง๋์ด๋ง 4์ฃผ์ฐจ ๊ณผ์ (1) | 2025.01.20 |
์ฐ๋ฆฌ FISA AI์์ง๋์ด๋ง 2์ฃผ์ฐจ ๊ณผ์ (0) | 2025.01.06 |
์ฐ๋ฆฌ FISA AI์์ง๋์ด๋ง 1์ฃผ์ฐจ ๊ณผ์ (0) | 2024.12.30 |